-
Notifications
You must be signed in to change notification settings - Fork 45.8k
New issue
Have a question about this project? Sign up for a free GitHub account to open an issue and contact its maintainers and the community.
By clicking “Sign up for GitHub”, you agree to our terms of service and privacy statement. We’ll occasionally send you account related emails.
Already on GitHub? Sign in to your account
[TF2 Object Detection] Converting SSD models into .tflite uint8 format #9371
Comments
Same problem here, triying now with different models of the model ZOO, for the moment impossible for me to convert to TFLITE a mobilenet V2 with TF2 Object detection API. |
You cannot directly convert the SavedModels from the detection zoo to TFLite, since it contains training parts of the graph that are not relevant to TFLite. Can you post the command you used to export the TFLite-friendly SavedModel using
Why unsure? What is the size of the converted model? You can use a tool like Netron to check if the .tflite model is valid. For quantization, you need to implement representative dataset gen in such a way that it mimics how you would typically pass image tensors to TFLite models. It usually boils down to something like this:
For the |
@srjoglekar246 Thanks for your reply.
For quantization, I thought it could be any data as long as it is image tensors, and thats why I used MNIST data which does not need to be downloaded. By the way, I think I saw somewhere that "representative_dataset_gen()" or TF2 takes images as numpy array, so could you let me know which is correct? I will try to do quantization following your suggested command line. I will update it here. Thanks. |
@srjoglekar246 Hi, I got this error while quantizing. Wonder if you are familiar with this kind of error. |
@srjoglekar246 Hi, I tried to train the model from a model zoo and convert it to .tflite and quantize it. However, there were 2 critical issues.
|
Not sure if there is a bug with training. The ODAPI folks might have a better idea of any issues that occur before using Can you show your exact code for tflite conversion? Though the model should probably be trained well first. |
@srjoglekar246 This is how I ran the step 1
and this is how I ran the step 2 and this is demo_convert
|
@SukyoungCho How did you run In your demo_convert, how are you implementing |
@srjoglekar246 sorry for confusing. this is how i ran
Yes, I ran about 12000 steps of training and tried converting it to tflite and quantize it. For preprocessing, |
@srjoglekar246 Should I implement preprocess within the script? like this?
|
@SukyoungCho Yes. |
@srjoglekar246 When I try to quantize the model, using the script above. It still gives me an error message below.. this is the exact script
|
This seems like an error with post-training quantization. Adding @jianlijianli who might be able to help. |
@SukyoungCho Can you confirm if conversion to float works? |
I remember it worked before. But, I will try it again and update it as soon as possible. |
@srjoglekar246 Hi, when I try conversion to float 32 or float 16, the code does not show any error and gives out a .tflite model file, but it is suspicious. The model produced by step 1 was about 7mb. However, the .tflite file from step 2 is only about 560 bytes - less than 1 kb. Here I attach the model resulted from the step 1 (https://github.com/tensorflow/models/blob/master/research/object_detection/g3doc/running_on_mobile_tf2.md) and the result from the step 2 (.tflite conversion to float 32). ssd_mobilenet_v2_fpnlite_320.zip Step 1 To use the script:
|
Hi! Try this solution (#9394 (comment)) It solve problem for me (and converting to INT too) |
Hi @mpa74, thank you so much for your help. I have tried the solution you offered and I was able to get .tflite file with seemingly legit size. However, I was not able to quantize it into In addition, when I perform step 1 and step 2, i am getting lots of error messages although it is giving out the converted file. So, I am not sure if they are converted properly. Could you let me know if you have experienced the same error message? Thank you so much. ##Error Message during step 1 (No Concrete functions found for untraced function)
##Errors during the step 2
|
Hi @SukyoungCho ! Looks like I have similar warnings on step 1, but error with ‘NoneType’ object I have never received. Sorry, I don’t know what to do with this error |
@mpa74 If a SavedModel is getting generated by step 1, the logs just seem to be warnings based on APIs etc. You can ignore them For step 2, you also need this for conversion:
The final post-processing op needs to run in float, there is no quantized version for it. |
@srjoglekar246 If you don't mind, could you elaborate more on what do you mean by "The final post-processing op needs to run in float, there is no quantized version for it." this? It sounds confusing to me, since the models from TF1 OD API are eligible for uint8 quantization, tflite conversion, and EdgeTPU compile while they have post processing ops on them. Is it due to a difference in tflite conversion processes for TF1 and TF2? To be honest, the reason why I was looking for EfficientDet uint8 was to deploy it on a board with coral accelerator. I have been using ssd mobiledet_dsp_coco from TF1 OD API, but its accuracy was not satisfactory (while i was only detecting 'person' the AP was about 38 and had way too many false positive). However, I found out that on the same image set, CenterNet and EfficientDet showed extremely low false positive rate (I ran an inference on the server for this not on the board). So it would be very appreciated if you could manage to release uint8 quantization for EfficientDet or CenterNet so that I can EdgeTPU compile it and deploy it on a board! Or, if you could tell me if there are any models better than SSD Mobiledet that can be quantized into uint8, or EfficientDet for TF1. Thank you so much! |
It looks like the Coral team has uncovered some issues with quantization of detection models. Will ping back once I have updates. |
Can you mention how you used |
oh my... thank you so much.... I just realized that my conversion batch script did not use the correct exporting script. So far things look more promising. model conversion now successfully terminates with the default settings and the interpreter of the resulting tflite model actually finishes the inference step.
are just |
The TF version you are using for conversion should be >=2.4. If an earlier version is used, the converter doesn't work with that the exporting script does. |
i am running this on tf version 2.5.0 |
Can you check the model size? If the conversion is happening incorrectly, thew model should be very small (few KB). |
so far integer quantization is not active. All still on default settings with float32 as datatype. tflite model file is currently on 440 bytes. That seems a bit small i guess? |
Yeah the converter doesn't seem to be working, and this was mainly observed when the converter was using an older version of TFLite. Can you try in a virtualenv with the latest TF? |
okay, thanks will try that. Will need some time to set that up though as i currently do not have anaconda installed. |
So i set up anaconda with python 3.9 and added tensorflow with all dependencies. I retrained my model over night on this fresh install and then did the checkpoint to saved_model conversion as well as the saved_model to tflite conversion from the py39 virtual env. The result remains the same with 440 bytes of model size. EDIT: EDIT2: while the model converted with i'll investigate this further to try and figure out why this happens. I assume that the latter version is the correct desired output. EDIT3: |
Yup :-) I wonder why your TFLite model isn't coming out right though. Can you try running this workflow in your environment? If the code in that Colab runs, then the problem is with what we are doing for your use-case. If the Colab also fails, the issue is probably with the system setup. |
i'll try but even the start is already a bit messy as it apparently is not made to run on python 3.9. EDIT: i guess skipping parts is not really possible as it all just builds on top of each other. |
Its mainly the TFLite section towards the end, instead of fine-tuning a model you can just use the SSD MobileNet downloaded from model zoo |
okay well that is intresting and confusing (at least to me) So from my understanding the code in python that i was trying to create to convert my saved_model to a tflite model was basically the way that the tflite_convert command uses just that it enables to option to add extra optimization steps as well as quantization. Is that correct? |
No, that model size doesn't sound right. AFAIK, it only happens when an older version of the converter doesn't know how to handle the TFLite-friendly SavedModel - as a result, it outputs a small model with just some zeros as outputs. Any converter after version 2.4 should be able to handle it, but in your setup for some reason it doesn't :-/ |
ah hold on i was just too stupid: its 10'000KB -> 10MB |
Oh I just saw your code carefully again, and looks like you are using |
ah yes that makes sense as i added this as a workaround that was needed for the wrongly created saved_model file. TL;DR for others up to here:
|
Nice! and happy to help :-) |
#!/usr/bin/python import numpy as np #path to images (100 sample images used for training) def representative_dataset_gen(): model_path = './exported-models/ptag-detector-model/ssd_mobilenet_v2_fpnlite_640x640/saved_model' #import trained model from mobilenet 640 v2 fpn converter = tf.lite.TFLiteConverter.from_saved_model(model_path) # using tensorflow converter.representative_dataset = representative_dataset_gen tflite_model_quant = converter.convert() interpreter = tf.lite.Interpreter(model_content=tflite_model_quant) with open('detect_quant.tflite', 'wb') as f: |
Hello, thanks for sharing code. I want to try but can you explain what is the "./quant-images/*.jpg" . I'm working with coco dataset. What ı will give there? Thanks. |
Since, I'm using a custom image set to train the folder point to the location of a my sample image set directory. In your case you can take a sample of 100 images from coco dataset and create a directory to convert. Just make sure you're setting the right dimension of the image you used for training since I'm using mobilenet v2 640x640 I'm resizing my images to that dimension. |
Thanks. When i try as you said, i'm getting this errror.
Traceback (most recent call last): ` |
@canseloguzz Can you try the instructions here to convert & run your model? Please try and see if the floating point conversion works fine first, then quantized. |
Hello @srjoglekar246 I have used this tutorial and tried to do the inference in android device it failed. Any workaround for this. I used I trained the model on my custom dataset. Exported the model using The command I used:
After exporting the model, I exported the TensorFlow Inference Graph
After this I converted the model into tflite
This is the floating point TF-Lite File. It's not working even after adding the metadata. I followed the steps mentioned here Even I used this Colab Notebook, used this model but model was crashing on my android device. I downloaded a model from TF-Hub to check my app it was working fine. I even used Netron to visualise the tflite file. There was a difference in both of them. The major difference is in the input arrays and output arrays. The TF-Hub model accepts input as But the tflite file which is converted doest not have this I also used this command to convert my model into tflite.
The tflite I got after conversion is 2KB and I have used neutron to view it does not have any layers. |
@sayannath You don't have you use |
@srjoglekar246 & Others, I have been trying to run inference after converting few models from TF2 obj detection zoo to TFLite using the process described in this guide, but getting wrong results from the tflite model (I am trying basic tflite model without doing any quantization or any other optimization as a first step). The models I have tried are: ssd_mobilenet_v2_320x320_coco17_tpu-8 The inference code I am using is similar to that posted by OP in this thread: #9287. Do we still need to export checkpoint using export_tflite_graph_tf2.py first and then convert resulting saved model to tflite(to be able to leverage various levels of quantization), or can we now (in April 2022) directly convert to tflite the saved model that gets downloaded when we download a model from TF2 detection zoo? Any help is appreciated. |
@SukyoungCho Were you able to successfully convert (by successfully convert, I mean not only able to convert to tflite models but also got decent/expected results) models from TF2 object detection zoo to tflite format while taking advantage of various types quantizations? Any help is appreciated. Thanks. |
@srjoglekar246 finally found a way to do it? seems that only @CaptainPineapple was successful |
Can you share the process @Petros626 ? |
@sayannath I have no process to share, only asking before I do the wrong thing. I converted in TF1 my model to a TFLite model successfully, but I know TensorFlow is sometimes difficult, so I wanted to ask first, if someone converted FPNLite 320x320 or 640x640 to a TFLite Model |
Hi, I was wondering if anyone could help how to convert and quantize SSD models on TF2 Object Detection Model Zoo.
It seems like there's a difference in converting to .tflite in TF1 and TF2. To the best of my knowledge, in TF1, we first frozen the model using exporter and then quantized and converted it into .tflite. And, I had no problem in doing it in TF1.
The models I tried was
However, when I followed the guideline provided on the github repo 1.(https://github.com/tensorflow/models/blob/master/research/object_detection/g3doc/running_on_mobile_tf2.md#step-1-export-tflite-inference-graph) and 2. (https://www.tensorflow.org/lite/performance/post_training_quantization#full_integer_quantization). I was not able to convert them into .tflite.
Running "Step 1: Export TFLite inference graph", created saved_model.pb file in the given output dir {inside ./saved_model/}
However, it displayed the skeptic messages below while exporting them, and not sure if it's run properly.
Running "Step 2: Convert to TFLite", is the pain in the ass. I managed to convert the model generated in the step 1 into .tflite without any quantization following the given command, although I am not sure if it can be deployed on the mobile devices.
But, I am trying to deploy it on the board with the coral accelerator and need to conver the model into 'uint8' format. I thought the models provided on the model zoo are not QAT trained, and hence they require PTQ. Using the command line below,
It shows the error message below, and i am not able to convert the model into .tflite format. I think the error occurs because something went wrong in the first step.
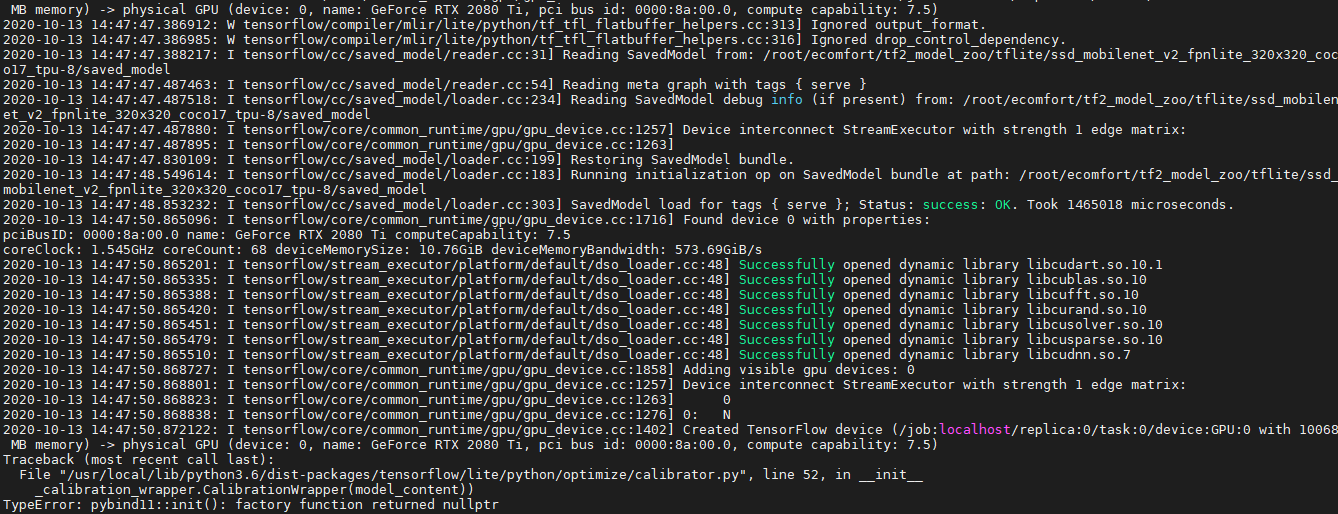
Below, I am attaching the sample script I used to run "Step 2". I have never train a model, and i am just trying to check if it is possible to convert SSD models on TF 2 OD API Model Zoo into Uint8 format .tflite. That is why, i dont have the sample data used to train the model, and just using MNIST data in Keras to save the time and cost to create data. (checkpoint CKPT = 0)
The environment description.
CUDA = 10.1
Tensorflow = 2.3, 2.2 (both are tried)
TensorRT =
ii libnvinfer-plugin6 6.0.1-1+cuda10.1 amd64 TensorRT plugin libraries
ii libnvinfer6 6.0.1-1+cuda10.1 amd64 TensorRT runtime libraries
It would be appreciated if anyone could help to solve the issue, or provide a guideline. @srjoglekar246 Would you be able to provide the guideline or help me to convert models into uint8? Hope you documented while you were enabling SSD models to be converted into .tflite. Thank you so much.
**_
_**
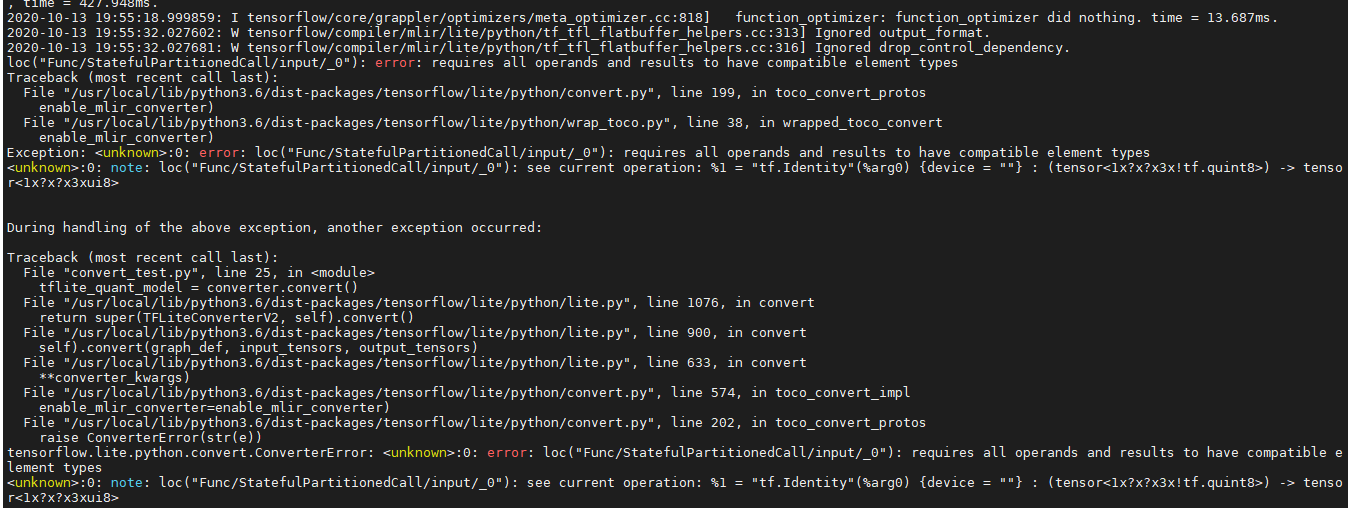
The text was updated successfully, but these errors were encountered: